Humanitarian OSM Team/top 10 data quality aspects
Background.
Even as OSM is rapidly growing in content and contributors, its credibility has been one of the main concerns for authoritative users. The belief that it is made by volunteers can limit the trust in the value of this free data source within traditional GIS communities. At HOT, we have prioritized the top 10 data quality aspects that we want to minimize. These aspects have been categorized under 3 categories are Positional Accuracy, Semantic Accuracy, and Completeness.
We came to reach the top 10 list through a number of consultations with the Data Quality Working Group, representatives from open mapping communities, and associates from HOT regional Hubs.
This information has been shared for reference by all OpenStreetMap data contributors, and users across the open mapping ecosystem, data quality associates at the HOT regional Hubs, HOT partners, and other communities that engage with OpenStreetMap.
HOT is focusing on prioritizing these aspects and implementation on how to minimize/eliminate them through HUB centered community engagements in form of trainings, collaborating with partners, and developing tools that can be used to improve the quality of mapping.
Top 10 data quality aspects.
There are several other issues affecting the quality of OSM data, however, our top 10 data quality aspects are;
- Spatial offsets.
- Temporal consistencies.
- Feature tracing inconsistencies.
- Road network consistency.
- Completeness of health facilities.
- Completeness of public service data for sustainable communities.
- Administrative boundary inconsistencies.
- Tagging.
- Logical consistencies of map features.
- Tasking Manager project consistencies.
Spatial Offsets:
An offset is the degree of deviation of an object from its intended position.
Category: Positional Accuracy
Possible Sources:
- OSM contributors that don’t recognize or know how to mitigate imagery offsets.
- New mappers that are not aware of the offsets.
- Different tile offsets in a given project on the TM.
- Use of different satellite images while mapping in the same vicinity.
- Low accuracy from mobile data collection tools as a result of obstacles while collecting data.
Examples of how we can address this:
- Strengthening and monitoring validation teams
- Extended training sessions on offsets for newcomers and validators.
- Stressing offsets in the instructions section of the TM
- Check the history of the existing feature and compare it with the different satellite images available.
- Advice mappers to remove offsets (especially JOSM editor users) when they exit a project that requires an offset
Rationale: - Spatial offset is one of the leading positional accuracy aspects that originate from the misalignment of satellite images that are used during desktop digitization that results from placing features at positions that deviate from their original.
- Spatial offsets result in overlapping features which affect the positional accuracy of OSM data. For example, buildings overlapping roads, public facilities in the middle of roads, and buildings in water bodies.
Temporal consistencies:
The inability to acquire the capture date of imagery or range is too broad to make capture metadata useful can lead to inconsistency in mapping.
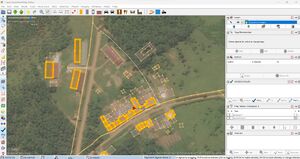
Category: Completeness
Possible Sources:
- Using imagery that is outdated - how can you identify what is the most recent imagery source?
- Data derived from AI/ML via feature extraction can be based on a mosaic of scenes with different capture dates. It can be very hard to verify the recency of data in these datasets (whether they’re buildings, roads, or something else)
- Data collected by mappers using survey tools can be seen as “wrong” or because it can’t yet be seen on imagery.
Examples on how we can address this:
- Developing training, guidance or technical solutions to imagery capture date determination (for project creation).
- Developing/delivering training for mappers to determine imagery recency.
- Work with imagery providers on technical solutions.
Rationale: - When outdated imagery is used, especially in areas where more recent mapping has occurred; mappers may delete valid data because it doesn’t appear in the imagery.
Feature tracing inconsistencies:
(overlapping buildings with buildings, buildings with highways, point features on buildings)
Overlapping features is a common aspect of data quality in OSM. The most visible aspect is when buildings overlap highways and compromise the spatial properties of these features. In practice, buildings should not overlap highways unless otherwise. In other cases, point features fall within the middle of highways.
Category: Positional Accuracy
Possible Sources:
- Offsets resulting from differences in satellite image alignments.
- Compromised accuracy from the data collected through mobile devices where the device experienced an obstacle during the data collection exercise. This causes point features to deviate from their expected positions.
- Differences in coordinate systems with data conflated from official sources. Different agencies collect data in different coordinate systems and in case of a conflation the conversion from UTM to WGS creates a shift in the position of the features on the ground.
- Overlapping data from duplicated efforts.
- Uploading AI-generated buildings without syncing them with the available OSM data.
- Low zoom levels in ID Editor where it shows no data mapped at that level.
- Blurry imagery in combination with beginner mappers
Examples of how we can address this:
- Correcting offsets before mapping new features.
- Data cleaning practices for mobile collected data before it is uploaded to OpenStreetMap.
- Following the corrected data conflation procedure while uploading AI-generated data.
- While mapping with ID Editor, make sure to zoom to the possible high level to be able to see if the features are mapped or not.
- Good initial training and good feedback from validators.
Rationale: - During validation, more time is wasted trying to correct the overlapping features.
- Building geometry inconsistencies affect the positional accuracy of buildings which can have an impact on assessing damage on buildings in case of disaster. Overlapping data reduces the geographical extent of the data and may result in wrong coverage analysis.
- Duplicated buildings and multiple buildings mapped one can mislead in the estimation of the number of households that may require relief in case of a disaster and the responding agency may derive wrong statistics from the data
Road network inconsistency:
(Segmented highways with inconsistent tags, hierarchy, outdated highway tags, and surfaces, hanging roads)
Category: Completeness
Possible Sources:
- Road mapping projects by beginner mappers with limited skill in snapping and road tagging.
- Tracing of roads at a low zoom level in Editing tools, especially ID Editor.
- The size of the projects and tasks can introduce errors, smaller projects can cause inconsistencies Size of tasks could mean nodes do not appear in a task and therefore will not be visible.
Examples of how we can address this:
- Training new mappers on the geometric properties of roads like connectedness.
- Clear instructions on the features to be mapped and emphasized by project managers.
- Experienced validation teams for road mapping projects.
- Training for Project Creators.
- Having local photos of areas to map can help improve - the wiki tool in JOSM allows you to bring in street view.
Rationale: - Broken roads pose a challenge during network analysis and road routing would be affected.
- With inconsistent roads, navigation using OSM navigation tools becomes difficult. Inconsistent highway tags can lead to poor planning of response routes, and the responding agency may end up using a longer route or a non motorable route.
Completeness of health facilities:
OpenStreetMap (OSM) data is collected by volunteer remote mappers around the world who at times may have limited resources to collect data on a large scope or even deploy surveys to collect attribute information about certain features. In the past 15 years, since the beginning of OSM, health facility data that volunteers produced has not been collected evenly and of today, most data is concentrated in urban areas thus large areas are still underrepresented and or the available datasets are not completely attributed.
Category: Completeness
Possible Sources:
- Not enough resources to cover big-scope health facility data collection projects.
- Mapping using mobile applications that allow limited attribution like Organic Maps, Maps.Me.
- Non standardized data models to be used during data collection activities.
Examples of how we can address this:
- Encourage public health-related projects in all OpenStreetMap communities through the Hubs.
- Standardize health data models to be used during data collection for health facilities.
- Ensure open participatory approaches for health data collection and mapping like including doctors and nurses in the project designs, special mapathons.
- Referring to healthsites.io for complete health facilities data model.
Rationale: - Health facilities are only concentrated in the urban areas leaving a gap for rural health facilities not mapped yet the entire public need to access health facilities be it urban or rural.
- A gap in the completeness of health facility data affects spatial analysis for where the services are located. Any person responding to a woman in labor may prefer to see the nearest health facility that offers maternity services.
- Also attribute completeness for example one would like to know the opening hours for a given health facility.
Completeness of public service data for sustainable communities:
Geographic and attribute data for public services in education, water points, and sanitation waste collection. As with health facilities, there has not been wide mapping projects that focus on these point features the way it has been for buildings and roads. The case of Uganda, detailed mapping of social facilities was done in the refugee camps in northern Uganda and also mapping for clean streets of Kampala where solid waste mapping was done in 2017. The remaining parts of the country have gaps in the social facility data
Category: Completeness
Possible Sources:
- Not enough resources to cover large scope mapping projects.
- Mapping using mobile applications that allow limited attribution like Organic Maps, Maps.Me.
Examples on how we can address this:
- Focusing on detailed point data collection beginning with cities, and municipalities then to other towns through activation of data collection campaigns.
- Considering available resources for data collection models for example Uganda Refugee mapping data model.
Rationale: - In relation to health facilities, other public social facilities are concentrated in either urban areas or in areas where HOT projects have taken place. There is a big gap in the distribution of mapped public facilities on OpenStreetMap.
- As with health facilities, there have not been wide mapping projects that focus on these point features the way it has been for buildings and roads. The case of Uganda, detailed mapping of social facilities was done in the refugee camps in northern Uganda, and also mapping for clean streets of Kampala where solid waste mapping was done in 2017. The remaining parts of the country have gaps in the social facility data.
Administrative boundaries:
Topological inconsistencies, broken relations, and outdated information.
Category: Completeness
Possible Sources:
- Administrative changes in the boundaries of districts and sub districts render the available data in OSM outdated.
- New mappers who may try mapping of boundaries in OSM and end up deleting boundary relations.
Examples on how we can address this:
- Coordinating with the agencies responsible for mapping boundaries on updating administrative boundaries in OSM.
Rationale: - Sometimes new countries are created, new districts are split and municipalities are being elevated to cities. As these changes happen, they should be also reflected on OpenStreetMap.
Tagging:
Objects in OSM are created by digitization and the attribution of tags. OSM does not provide a rigorous classification system of the geographical objects. It just gives some recommendations and a set of predefined tags that can be used to define the objects. Thus, the final description attributed to the objects is defined by the mappers based on their knowledge about the object. This can lead to incorrect tag definitions since sometimes it is difficult for new mappers to differentiate between objects that fall in similar classes
Category: Semantic
Possible Sources:
- Misspelled tag values or capitalization of tags by experienced mappers who know what to do but are careless in application of tags.
- Uploading information to OSM without undergoing a data cleaning and conflation process.
Examples of how we can address this:
- Training new mappers on tagging and giving them access to all available OSM tagging resources.
- Discourage uploading non official data to OSM.
Rationale:
- Tag information can affect decision-making where an agency is relying on numbers to inform decision.
- An indoor corridor wrongly tagged as a tunnel might be calculated as a shortest path by navigators and routing applications like PG Routing.
Logical consistencies of map features:
There are map features that are positionally related to others and must be located with on or near other features.
Category: Positional Accuracy
Possible Sources:
- Wrong tagging of features by beginner mappers for example tagging a railway station as a bus stop.
- Uploading data collected from the field without cleaning it and checking the GPS position of the data.
- Shift caused by differences in the coordinate systems.
- Mapping/tracing features at a low zoom level.
Examples of how we can address this:
- Using a standardized coordinate system for data collection that is similar to the OpenStreetMap coordinate system.
- Developing training materials for new mappers to guide them on OSM tagging.
Rationale: - Logically there are points that must be inside buildings. They include cafes, schools, pharmacies, and supermarkets, which should be located within the building polygons.
- Points that are semantically related to the road network and must be outside the road like bus stops, parking, and street lamps, traffic lights which are related to the roads and are usually located very close to them but not on them but which should not be located within buildings.
Tasking Manager project consistencies:
Issues with data quality can be directly derived from inconsistencies relating to the quality of projects created on Tasking Manager
Category: Semantic
Possible Sources:
- Tasking Manager Projects themselves can be a source of errors. These include errors from overlapping projects, unclear instructions on what should be mapped and what shouldn't be mapped, and the level of difficulty.
- Projects with dense existing mapped data can be compromised if the level of difficulty is set to beginner. In a building mapping project, roads should not be mapped because it may result in hanging roads mapped in one task and not in the adjacent tasks.
- Unresponsive project managers leaving critical questions unanswered
Examples of how we can address this:
- Ensuring all project creators have an appropriate understanding of good project creation and management.
- Requiring, and delivering, a minimum level of participation and refresher training for all project creators.
- Tasking Manager project instruction template with project info on fixed places: e.g. first required imagery, second required mappings, third yes or no offset etc.
Rationale: Projects can be troublesome for mappers (and validators) for many reasons, such as:
- Permissions and/or difficulty not appropriate for the actual difficulty of mapping.
- Instructions not complete, confusing, misleading or just ‘too much text’ for mappers to follow.
- Wrong or not the most appropriate imagery set as default.
- Asking for too many, or dissimilar features; and/or task size not appropriate for features requested.